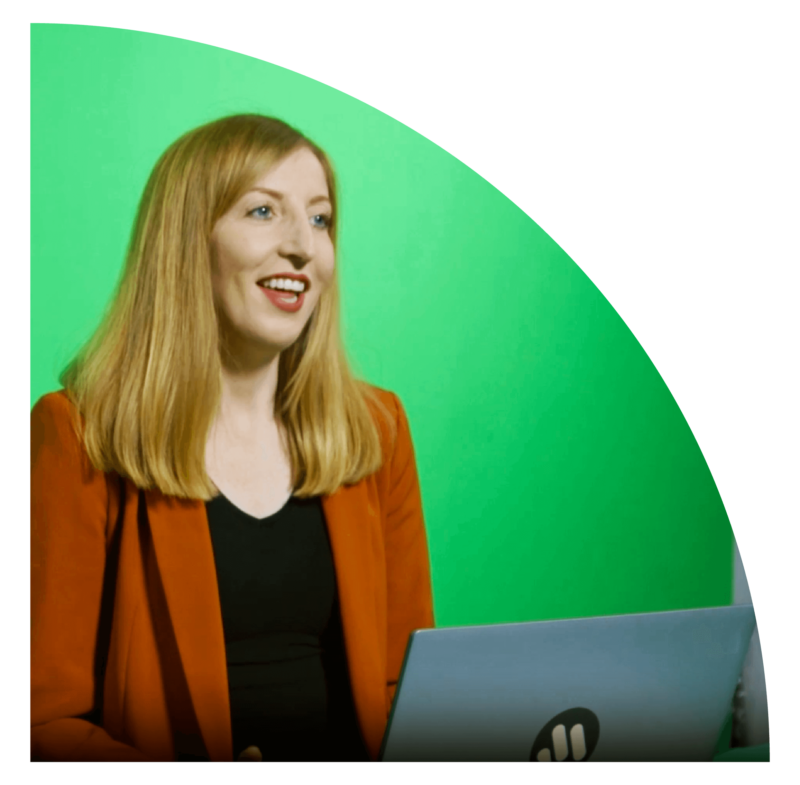
Return on eXpertise
Gain access to essential insights and content.Valuable real-life, real-time insights through a variety of channels, including blogs and podcasts. For more in-depth research, there are a variety of resources, such as eBooks, reports, and white papers that enable you to outpace the speed of change.Through original research and analysis, we provide unique insights and perspectives on the latest developments. We are not bystanders, we are active participants in shaping the future, sharing our expertise and driving meaningful discussions in your industry.
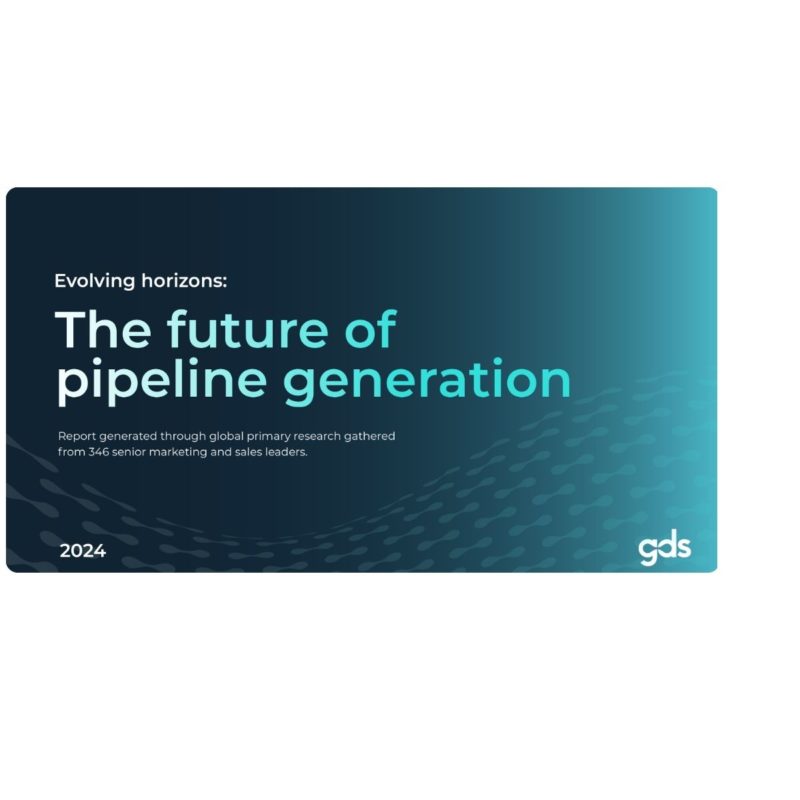
Evolving horizons:
The future of pipeline generation
Unlock the secrets of 346 senior sales and marketing leaders to elevate your 2024 strategies. Unlock insights into evolving budgets, shifting priorities, and key growth areas.
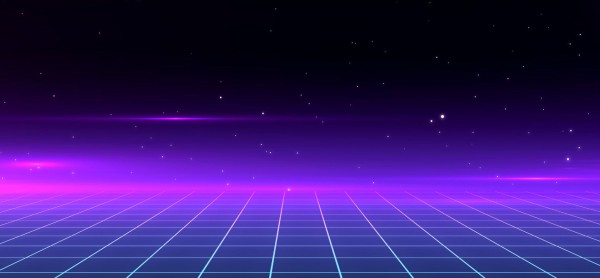
How SundaySky used events to generate 15 follow-up meetings and over $500k in attributable pipeline
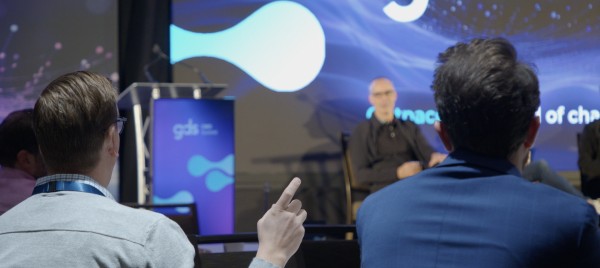
Top 3 healthcare technology trends in 2024
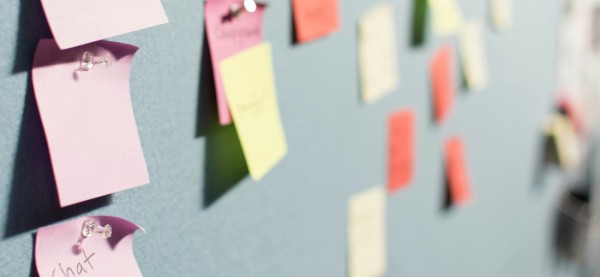
Guiding top executives on a strategic path towards success
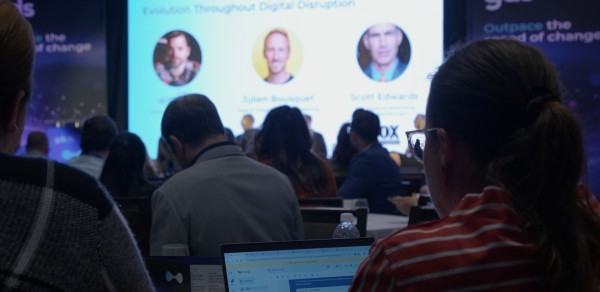
The shift from AI ideation to AI adoption
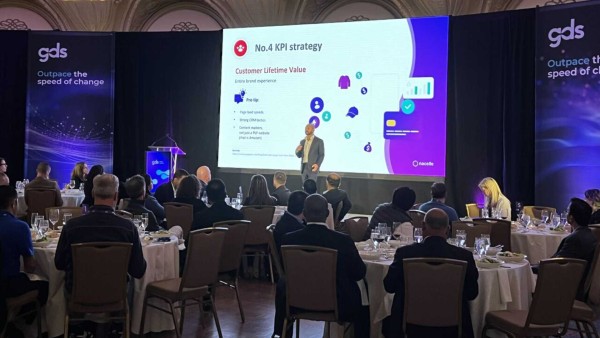
3 must-know retail predictions from industry innovators
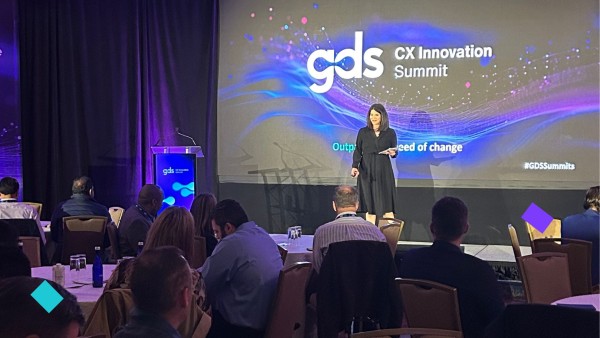
Top insider secrets from CX leaders
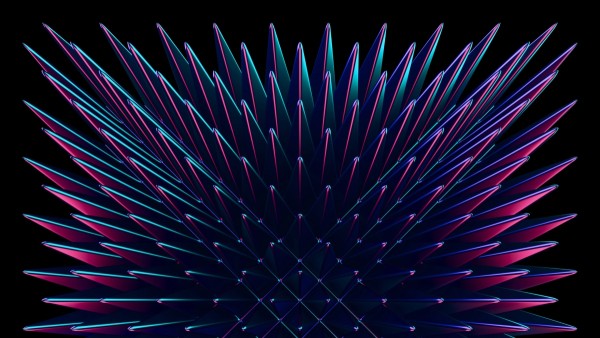
Adding AI to the CMO Swiss Army Knife
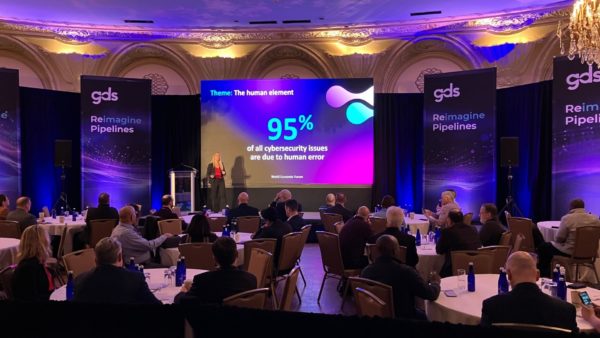
Key findings from the GDS Security Insight Summit North America
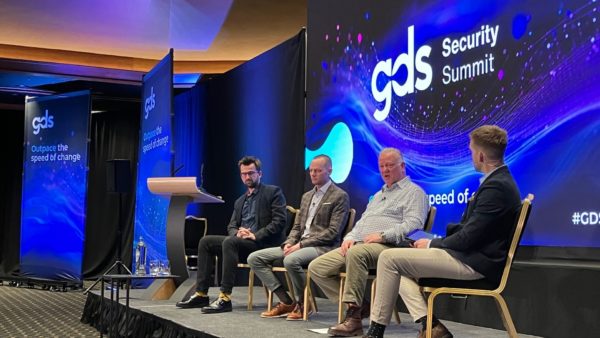
3 key takeaways from the GDS Security Insight Summit EU
Get in touch today
Fill out the form below and one of our team will get back to you right away to discuss how we can help you.
f27cee47-6a35-46b3-aca5-f35ee4851558
Frequently asked questions
We are a global B2B solution provider, specializing in helping businesses connect with their desired audiences to drive higher return on expectations for pipeline growth and transformation.
With 30 years of expertise, we’ve become our clients’ pipeline partner, providing real-life, real-time insights through innovative virtual and face-to-face environments.
Leading brands trust us to deliver pioneering immersive experiences, unparalleled access to industry thought leadership and unique creative content and production services to help solve challenges and accelerate their projects.
GDS Group delivers pioneering immersive experiences, such as Digital Summits, Digital Showcases, Digital Roundtables, and In-person Experiences.
There are a couple of ways you can participate in a GDS event experience; as a partner or as an attendee. Whichever you are interested in, do get in touch
Yes! We partner with leading brands all across the globe – for partnership opportunities please get in touch